Edge ML
In an era defined by the vast generation of data, the demand for efficient processing and real-time decision-making has never been greater. Traditional methods of centralized data processing, while effective, often encounter bottlenecks due to latency, bandwidth limitations, and privacy concerns. Enter edge machine learning, a paradigm-shifting approach that distributes computational tasks to the network's periphery. Edge ML allows for rapid data analysis and decision-making directly at the source. In this article we'll take a look at the world of edge machine learning, exploring its principles, applications, challenges, and the transformative impact ML at the edge holds across various domains.
Understanding Edge Machine Learning
Edge machine learning refers to the deployment of machine learning algorithms and models directly on edge devices or gateway hardware, rather than relying solely on centralized cloud servers for data processing. These edge devices include smartphones, IoT sensors, drones, autonomous vehicles, and other endpoints that collect data at the edge of the network.
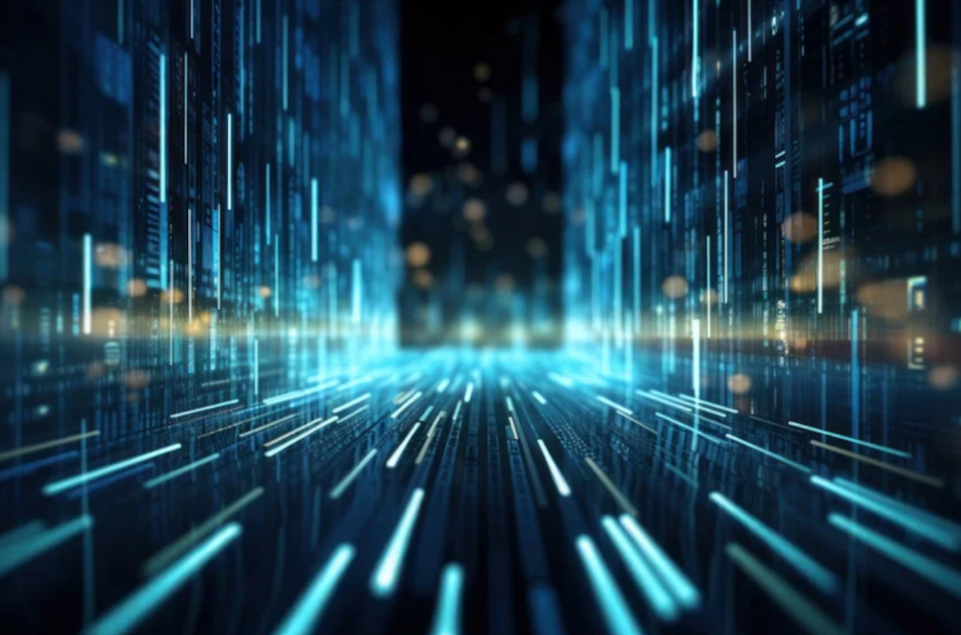
Machine Learning & Edge Computing
The integration of machine learning and edge computing is revolutionizing how we process and analyze data in real-time. This combination addresses two critical challenges in modern computing: the need for rapid decision-making and the limitations of network bandwidth.
The primary objective of edge machine learning is to reduce latency and bandwidth usage by performing data analysis and inference locally. This approach marks a departure from traditional cloud-based ML models, where data is sent to centralized servers for processing.
By processing data at the edge, organizations can achieve real-time insights and decision-making, enhancing operational efficiency and enabling rapid responses to dynamic environments.
Several key benefits can be achieved by processing at the edge:
However, implementing edge ML comes with its own set of hurdles. The limited computational power of edge devices compared to cloud servers means that ML models need to be optimized for efficiency without sacrificing accuracy. This has led to innovations in model compression techniques and the development of specialized hardware for edge AI.
Companies like NVIDIA, Intel, and Google are at the forefront of developing edge AI hardware, creating powerful yet energy-efficient chips designed specifically for running ML models on edge devices. These advancements are enabling more complex AI applications to run at the edge, from computer vision in security cameras to natural language processing in smart home devices.
Applications Across Industries
Edge machine learning is transforming various sectors by enabling real-time data processing and decision-making at the point of data generation. Here's a look at its applications across different industries:Healthcare
In healthcare, edge machine learning facilitates remote patient monitoring, predictive maintenance of medical equipment, and personalized treatment recommendations. Wearable devices equipped with edge ML capabilities can continuously monitor vital signs, detect anomalies, and alert medical professionals in case of emergencies, thereby improving patient outcomes and reducing healthcare costs. In healthcare, edge machine learning is revolutionizing patient care and operational efficiency.
Manufacturing
Edge machine learning revolutionizes manufacturing processes by enabling predictive maintenance, quality control, and real-time optimization of production lines. By analyzing sensor data from machinery and equipment at the edge, manufacturers can identify potential faults before they lead to downtime, optimize resource utilization, and ensure product quality consistency. Edge machine learning is transforming manufacturing processes, improving efficiency and product quality.
Autonomous Vehicles
Edge machine learning plays a crucial role in the development of autonomous vehicles, where split-second decision-making is paramount for ensuring passenger safety. By deploying ML models directly on onboard processors, vehicles can interpret sensor data in real-time, detect and classify objects, anticipate hazards, and navigate complex environments autonomously. Edge machine learning is crucial for the development and operation of autonomous vehicles.
Retail
In the retail sector, edge machine learning powers personalized customer experiences, inventory management, and demand forecasting. Smart shelves equipped with edge ML capabilities can track inventory levels, analyze customer behavior, and deliver targeted promotions in real-time, enhancing customer satisfaction and optimizing sales. In retail, edge machine learning is enhancing customer experiences and operational efficiency.
Edge machine learning is where intelligence meets immediacy, transforming data into action at the speed of relevance.
EdgeML Challenges and Considerations
While edge machine learning offers numerous benefits, its implementation comes with several challenges and considerations:
Resource Constraints - Edge devices often have limited computational power, memory, and battery life, posing challenges for deploying complex machine learning models. Optimizing models for efficiency and scalability is essential to ensure optimal performance at the edge.
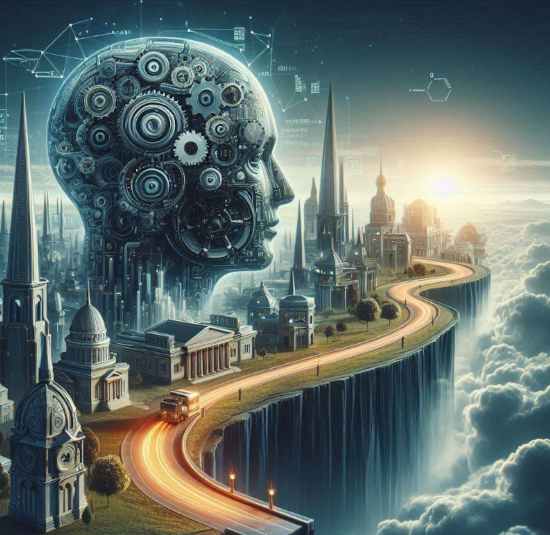
Data Security and Privacy - Edge devices capture sensitive data that must be processed and analyzed securely to prevent unauthorized access and privacy breaches. Implementing robust encryption, authentication, and access control mechanisms is crucial to safeguarding data integrity and confidentiality.
Model Updates and Maintenance - Managing and updating machine learning models deployed at the edge pose logistical challenges, especially in large-scale deployments with diverse edge devices. Establishing efficient mechanisms for remote model updates, version control, and performance monitoring is essential to ensuring model accuracy and reliability over time.
Interoperability and Standards - The heterogeneity of edge devices and platforms complicates interoperability and standardization efforts, hindering seamless integration and collaboration across different vendors and ecosystems. Establishing industry-wide standards and protocols is essential to promote interoperability and facilitate the development of interoperable edge ML solutions.
Future Perspectives
As edge computing and machine learning technologies continue to advance, the future of edge machine learning appears promising. Emerging trends such as federated learning, which enables collaborative model training across distributed edge devices while preserving data privacy, hold immense potential for unlocking new capabilities and applications at the edge.
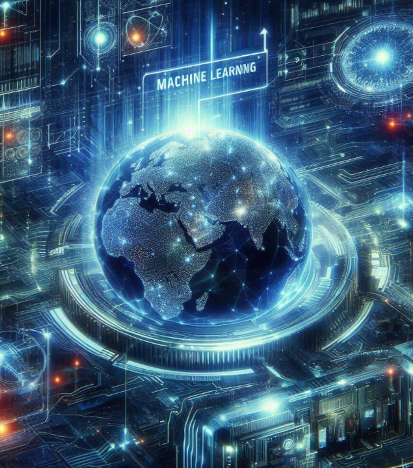
Advancements in hardware accelerators, such as specialized AI chips and neuromorphic processors, are poised to enhance the performance and energy efficiency of edge ML inferencing, enabling more sophisticated applications in resource-constrained environments.
Edge machine learning represents a transformative shift in data processing and decision-making, empowering organizations to harness the full potential of data at the network's edge. By distributing computational tasks to edge devices, organizations can achieve real-time insights, improve operational efficiency, and enable innovative applications across various industries.
Realizing the full potential of edge machine learning requires addressing technical challenges, ensuring data security and privacy, and fostering interoperability and collaboration across diverse edge ecosystems.
With continued advancements in edge computing and machine learning technologies, the future of edge machine learning holds immense promise for revolutionizing how we process data and make decisions in an increasingly connected world.
Learn more about Edge ML here: Edge ML Overview.
Machine Learning Artificial Intelligence News
https://machinelearningartificialintelligence.com
AI & ML
Sign Up for Our Newsletter