Embracing the Essence of Computational Artificial Intelligence
Computational intelligence (CI) or Computational Artificial Intelligence (Computational AI) is a multifaceted domain that encompasses the ability of computers to learn tasks from data or observation, mirroring the cognitive processes of humans.
Often equated with soft computing, CI defies a universal definition, yet it stands as a beacon of innovation in addressing complex real-world problems where traditional mathematical modeling falls short. The allure of computational intelligence lies in its emulation of human-like reasoning, adaptive control, and the ability to navigate uncertainties inherent in many real-life scenarios.
Unveiling the Pillars of Computational Intelligence
Computational intelligence draws upon a repertoire of nature-inspired computational methodologies, each playing a pivotal role in unraveling the intricacies of complex systems. At its core are five complementary techniques: fuzzy logic, artificial neural networks, evolutionary computing, learning theory, and probabilistic methods. These techniques enable machines to grapple with inexact and incomplete knowledge, fostering adaptability and fault tolerance akin to human cognition.
These techniques collectively enable CI systems to adapt, learn, and solve problems in ways that more closely resemble human cognitive processes. So how is CI different from AI? Unlike traditional AI approaches that rely heavily on hard computing and binary logic, CI embraces soft computing methods that can handle the nuances and complexities of real-world scenarios.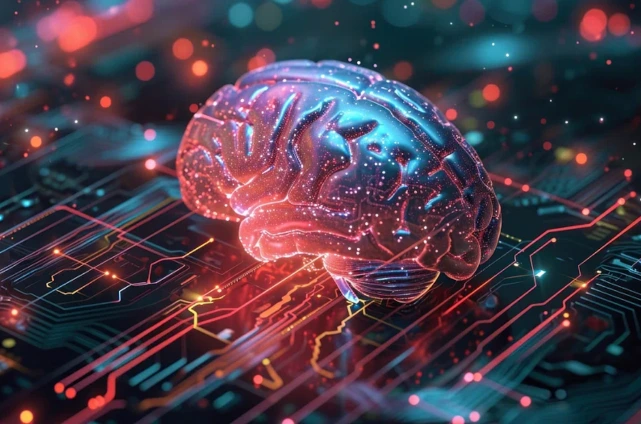
Computational AI Techniques
Fuzzy Logic
Fuzzy logic empowers computers to comprehend natural language and tackle real-world processes characterized by uncertainty and imprecision. Its applications span diverse domains, from control systems and image processing to medical diagnostics and business strategy selection. Unlike traditional AI, which demands precise data, fuzzy logic thrives on approximate reasoning, offering a pragmatic solution to complex problems.
Artificial Neural Networks
Modeled after biological neural networks, artificial neural networks embody distributed information processing systems capable of learning from experiential data. With fault tolerance as a hallmark feature, neural networks excel in data analysis, classification, associative memory, and pattern generation. Their versatility extends to medical diagnostics, fraud detection, and nonlinear system control, aligning closely with human-like learning mechanisms.
Computational intelligence is the bridge between human ingenuity and machine capability, forging a path towards a future where intelligent systems empower humanity to overcome the most complex challenges of our time.
Evolutionary Computation
Inspired by biological evolution, evolutionary computation encompasses a suite of algorithms for global optimization. Genetic algorithms, evolution strategies, and genetic programming are among the arsenal of tools employed to tackle optimization challenges unsolvable by traditional mathematical methods. Evolutionary computation shines in multi-objective optimization scenarios, paving the way for innovative problem-solving approaches.
Learning Theory
Learning theory delves into the cognitive processes underpinning human learning, elucidating how experiences shape knowledge acquisition and decision-making. By leveraging insights from psychology, learning theory enables computational systems to discern patterns, make predictions, and refine behaviors based on past experiences. It serves as a cornerstone for building adaptive and intelligent systems that mimic human learning mechanisms.
Probabilistic Methods
Rooted in fuzzy logic, probabilistic methods offer a framework for evaluating outcomes in computational intelligence systems characterized by randomness. By harnessing prior knowledge and assessing uncertainties, probabilistic methods unravel potential solutions to complex problems. From risk assessment to decision-making, probabilistic methods provide invaluable insights into navigating uncertain environments.
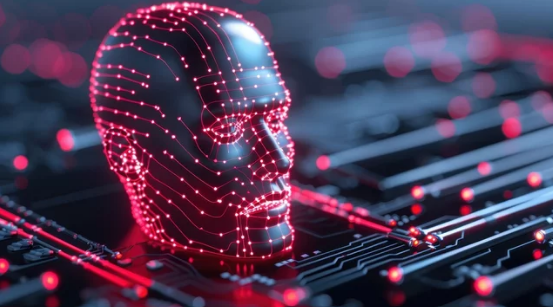
Navigating the Landscape of CI vs AI
While computational intelligence shares common goals with Artificial Intelligence (AI), it distinguishes itself through its reliance on soft computing methods and adaptability to diverse situations.
Unlike hard computing techniques that adhere to binary logic, soft computing techniques, such as fuzzy logic, embrace the nuances and partial truths inherent in real-world scenarios. This fundamental difference shapes how each approach tackles complex problems and interacts with real-world data.
Key Distinctions:
1. Approach to Uncertainty: AI: Traditional AI often relies on hard computing techniques that work with precise, deterministic data and well-defined rules. CI: Embraces uncertainty and imprecision, using soft computing methods to handle ambiguous or incomplete information.2. Logic Systems: AI: Typically uses binary logic (true/false, 0/1) as the foundation for decision-making processes. CI: Employs fuzzy logic, allowing for degrees of truth and partial membership in sets, more closely mimicking human reasoning.
3. Adaptability: AI: Often requires extensive training on large datasets and may struggle with novel situations outside its training scope. CI: Designed to adapt to changing environments and learn from ongoing interactions, making it more flexible in real-world applications.
4. Problem-Solving Approach: AI: Tends to excel at well-defined problems with clear objectives and constraints. CI: Better suited for complex, ill-defined problems where traditional mathematical modeling falls short.
5. Learning Mechanisms: AI: Relies heavily on machine learning algorithms, often requiring large amounts of labeled data for supervised learning. CI: Incorporates a broader range of learning techniques, including evolutionary algorithms and neural networks that can learn from less structured data.
6. Interpretability: AI: Some AI models, particularly deep learning systems, can be "black boxes" with decision-making processes that are difficult to interpret. CI: Often provides more transparent decision-making processes, especially in fuzzy systems where rules can be more easily understood and modified.
7. Biological Inspiration: AI: While some AI techniques are inspired by biological processes, many are purely mathematical or statistical in nature. CI: More directly inspired by biological systems, incorporating concepts from neural networks, evolutionary processes, and swarm intelligence.
8. Handling of Partial Truths: AI: Often struggles with partial truths or ambiguous information, requiring clear-cut categorizations. CI: Excels in handling partial truths through fuzzy logic, allowing for more nuanced decision-making.
9. Robustness: AI: Can be sensitive to noise in data and may require extensive data cleaning and preprocessing. CI: Generally more robust to noisy or imperfect data, making it suitable for real-world applications where data quality varies.
10. Application Domains: AI: Widely applied in areas like natural language processing, computer vision, and strategic game-playing. CI: Particularly strong in control systems, pattern recognition, optimization problems, and decision support systems.
In practice, the boundaries between AI and CI are often blurred, with many modern systems incorporating elements of both approaches. The choice between AI and CI techniques often depends on the specific problem at hand, the available data, and the desired outcomes. As the field of artificial intelligence continues to evolve, we're likely to see further integration of these approaches, leveraging the strengths of both to create more powerful and adaptable intelligent systems.
Tracing the Evolution of Computational Intelligence
The roots of computational intelligence can be traced back to the early 1990s, with the IEEE Neural Networks Council laying the groundwork for its emergence. Over time, the field evolved to encompass a broader spectrum of interests, including fuzzy systems and evolutionary computation.Bezdek's seminal work in 1994 provided a definitive definition of computational intelligence, underscoring its focus on low-level data processing, pattern recognition, and adaptive computation.
As the field matured, it began to incorporate additional nature-inspired methodologies, such as swarm intelligence and artificial immune systems. These developments expanded the toolkit of computational intelligence, enabling it to tackle increasingly complex real-world problems. The late 1990s and early 2000s saw a surge in research and applications, with computational intelligence techniques being applied to diverse domains such as finance, robotics, and bioinformatics. This period also witnessed the integration of computational intelligence with other emerging fields, such as data mining and machine learning, further enhancing its capabilities and relevance in the rapidly evolving landscape of artificial intelligence.
Bridging the Divide: Computational Intelligence vs. Traditional AI
While computational intelligence falls under the umbrella of artificial intelligence, it diverges from traditional AI in its reliance on soft computing techniques and adaptability to uncertain environments. Where hard computing techniques falter in translating natural language and handling imprecise data, soft computing techniques excel, offering a more nuanced and human-like approach to problem-solving.
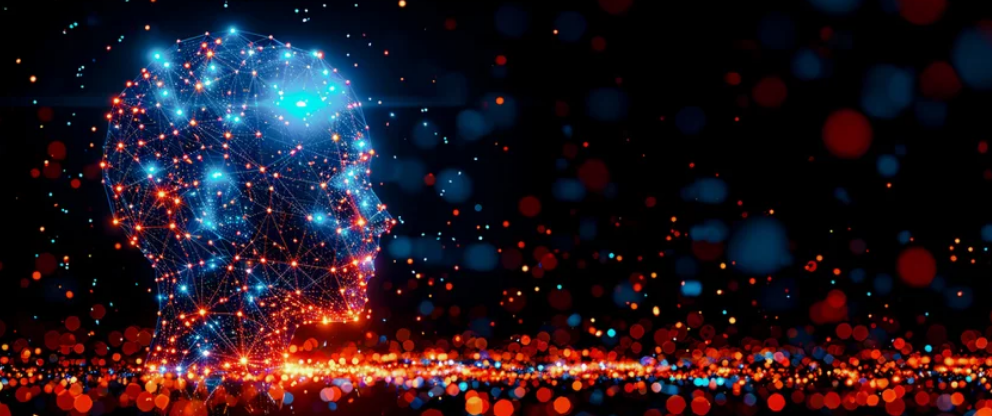
Embracing the Future of Computational Intelligence
As the landscape of artificial intelligence continues to evolve, computational intelligence stands poised at the forefront of innovation, offering novel solutions to complex real-world problems.
By harnessing the power of fuzzy logic, artificial neural networks, evolutionary computation, learning theory, and probabilistic methods, computational intelligence heralds a future where machines seamlessly collaborate with humans to tackle the most pressing challenges of our time.
Computational intelligence represents a paradigm shift in the way we approach problem-solving, bridging the gap between human cognition and machine intelligence. With its diverse array of techniques and applications, computational intelligence offers a glimpse into a future where intelligent systems coexist harmoniously with human endeavors, enriching our lives and propelling us towards new frontiers of discovery and innovation.
Machine Learning Artificial Intelligence News
https://machinelearningartificialintelligence.com
AI & ML
Sign Up for Our Newsletter