Deep Learning: The Powerhouse of Modern AI
What is Deep Learning?
Deep learning is a technique within artificial intelligence (AI) that trains computers to interpret data in a manner inspired by the human brain. Deep learning systems can identify intricate patterns in images, text, audio, and other data to generate precise insights and predictions. These techniques can automate tasks that usually necessitate human intelligence, such as describing visuals or converting audio files into text.
Why is Deep Learning Important?
AI endeavors to train computers to think and learn like humans. Deep learning underpins numerous AI applications found in everyday products, including:
Moreover, it is vital for advancing technologies like self-driving cars, virtual reality, and beyond.
Deep learning models are digital files that data scientists have trained to execute tasks using algorithms or predetermined sequences of steps. Companies leverage these models to analyze data and make predictions across various applications.
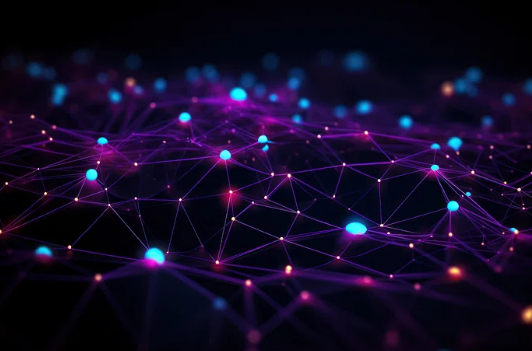
What are the Uses of Deep Learning?
Deep learning is employed in numerous fields such as automotive, aerospace, manufacturing, electronics, medical research, and more. Here are some examples:
Autonomous Vehicles and Deep Learning
Autonomous vehicles rely heavily on deep learning to navigate and interact with their environment safely. By utilizing deep learning, these vehicles can accurately identify road signs, pedestrians, and other crucial elements on the road.Due to its dynamic nature, AI continuously works to reduce false positives (blocking legitimate users) by refining its accuracy. It operates at such speeds that it does not affect the user experience. The top AI cybersecurity solutions are lightweight, ensuring they don’t impact the performance of your website or mobile app.
Deep learning enables autonomous vehicles to:
By continually learning from new data, deep learning models improve their accuracy and reliability over time. This capability is crucial for the development of safe and efficient autonomous vehicles, as it ensures they can adapt to various driving conditions and environments.
Defense Systems and Deep Learning
Defense systems increasingly rely on deep learning to enhance their capabilities in identifying significant areas within satellite images. By leveraging deep learning, these systems can efficiently analyze vast amounts of data and pinpoint crucial details that might be otherwise overlooked.Deep learning enables defense systems to:
Through continuous learning and adaptation, deep learning models become more effective at identifying significant patterns and anomalies in satellite imagery. This capability significantly enhances the strategic and tactical decision-making processes in defense systems, providing a robust tool for national security and intelligence efforts.
Medical Imaging and Deep Learning
Medical imaging has been revolutionized by deep learning, particularly in the detection and diagnosis of cancer cells. By employing deep learning techniques, healthcare professionals can achieve more accurate and efficient diagnoses, ultimately improving patient outcomes.Deep learning enables medical imaging to:
Through continuous learning and adaptation, deep learning models improve their diagnostic capabilities over time. This ongoing improvement is crucial for staying ahead of the evolving nature of cancer and other diseases. The integration of deep learning in medical imaging represents a significant advancement in the fight against cancer, providing a powerful tool for early detection and precise diagnosis.
Factories and Deep Learning
Factories are increasingly utilizing deep learning to enhance safety and operational efficiency by recognizing when people or objects are too close to machinery. This application of deep learning helps prevent accidents and ensures a safer working environment.Deep learning enables factories to:
Retail and Popular Voice Assistants
Voice assistants are extremely popular today. Everyone is familiar with Google Assistant, Alexa, and Siri. These assistants listen to human commands through microphones and perform tasks such as ordering goods online or scheduling appointments.Predictive Advertising
Predictive advertising leverages data to revolutionize marketing efforts, transforming creativity and brainstorming into high-revenue activities. At the core of this approach is the collection and analysis of customer, prospect, market, and competitor data. This encompasses behavioral data, purchasing journeys, transactions, search histories, and more.By utilizing this data, predictive advertising forecasts which audiences are most likely to engage with an ad. Advertisers can then precisely target these audiences and optimize their ad copies and budget allocations. The contribution of deep learning (DL) to successful campaigns is immense. DL processes vast amounts of product images, texts, websites, and technical details of each user, ensuring campaigns are targeted with exceptional accuracy and effectiveness
Through continuous learning, deep learning models become better at detecting potential hazards and improving workplace safety. The integration of deep learning in factory environments not only minimizes the risk of accidents but also promotes a culture of safety and vigilance among workers, leading to a more secure and productive workplace.
These use cases and many more like them fall into four broad categories: computer vision, speech recognition, natural language processing (NLP), and recommendation systems.
Computer Vision
Computer vision enables computers to derive information and insights from images and videos. Deep learning allows computers to understand visuals similarly to humans. Applications include:Speech Recognition
Deep learning models can analyze human speech despite variations in patterns, pitch, tone, language, and accent. Virtual assistants like Amazon Alexa and transcription software use speech recognition to:Natural Language Processing
Using deep learning algorithms, computers can extract insights and meaning from text and documents. This capability has various applications, including:Recommendation Engines
Applications can utilize deep learning to monitor user activity and provide personalized recommendations. By analyzing user behavior, they help users discover new products or services. For instance, media and entertainment companies like YouTube, Fox, and Peacock use deep learning to offer personalized video suggestions.Learn more about AI and ML here: ML AI News.
Machine Learning Artificial Intelligence News
https://machinelearningartificialintelligence.com
AI & ML
Sign Up for Our Newsletter