AI-Powered Fraud Detection: Revolutionizing Security in the Digital Age
Innovative AI Strategies for Identifying and Mitigating Financial Fraud Risks
1.1 Background
The financial services industry is experiencing a rapid digital transformation, with online transactions, mobile banking, and digital payments becoming ubiquitous. This shift has brought unprecedented convenience to consumers but has also opened new avenues for fraudulent activities. According to the Association of Certified Fraud Examiners (ACFE), organizations lose an estimated 5% of their annual revenues to fraud (ACFE, 2022). The complexity and speed of modern fraud schemes have rendered traditional rule-based detection systems obsolete, necessitating more sophisticated approaches.1.2 Research Objectives
This study aims to: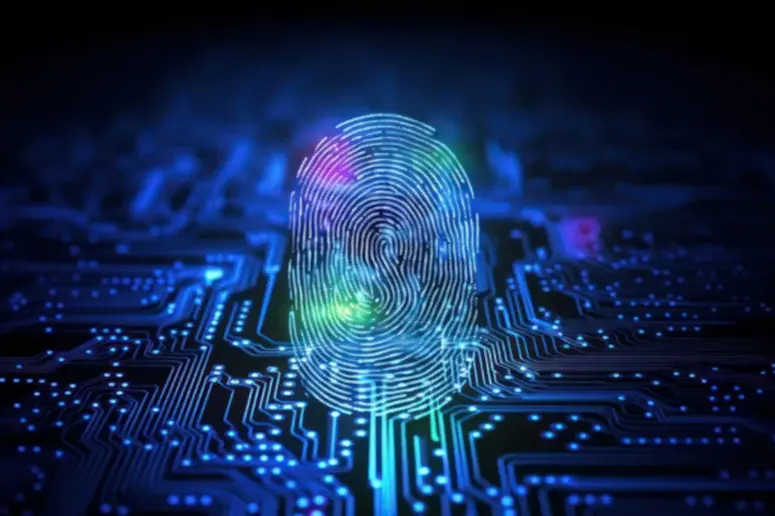
1.3 Methodology
Our research methodology combines:2.1 Types of Financial Fraud
Financial fraud encompasses a wide range of illicit activities, including:a) Credit card fraud:
b) Identity theft:
c) Money laundering:
d) Synthetic identity fraud:
e) Insider trading:
f) Insurance fraud:
2.2 Emerging Fraud Techniques
Cybercriminals are continuously developing new methods to exploit vulnerabilities in financial systems. Some emerging techniques include:a) Deepfake technology for identity fraud:
b) AI-generated phishing attacks:
c) Adversarial machine learning to evade detection:
d) Cryptocurrency-based money laundering schemes:
e) Social engineering tactics:
f) IoT-based fraud:
2.3 Limitations of Traditional Fraud Detection Methods
Traditional fraud detection methods, such as rule-based systems and statistical models, suffer from several limitations:a) Inability to adapt quickly to new fraud patterns:
b) High false positive rates, leading to customer friction:
c) Limited capacity to process large volumes of data in real-time:
d) Difficulty in detecting complex, multi-dimensional fraud schemes:
e) Lack of holistic view:
f) Scalability issues:
3.1 Machine Learning Algorithms
Machine learning algorithms form the backbone of modern fraud detection systems. These algorithms can be broadly categorized into supervised, unsupervised, and semi-supervised learning approaches.3.1.1 Supervised Learning
Supervised learning algorithms are trained on labeled datasets where the outcome (fraudulent or legitimate) is known. These algorithms learn to classify new, unseen data based on patterns observed in the training data.a) Random Forests
Random Forests are ensemble learning methods that construct multiple decision trees during training. In fraud detection, they excel at handling high-dimensional data and can capture complex interactions between features.
Example application: A study by Bhattacharyya et al. (2011) demonstrated that Random Forests outperformed other classifiers in detecting credit card fraud, achieving an AUC (Area Under the Curve) of 0.942.
b) Support Vector Machines (SVM)
SVMs are powerful classifiers that find the optimal hyperplane to separate classes in high-dimensional space. They are particularly effective when dealing with non-linearly separable data through the use of kernel functions.
Example application: Research by Sahin and Duman (2011) showed that SVMs achieved a 99% accuracy rate in detecting credit card fraud when combined with feature selection techniques.
c) Gradient Boosting Machines (GBM)
GBMs, including algorithms like XGBoost and LightGBM, build an ensemble of weak learners (typically decision trees) in a stage-wise manner. They are known for their high performance and ability to handle imbalanced datasets, which is common in fraud detection scenarios.
Example application: A study by Zhang et al. (2018) found that XGBoost outperformed other machine learning algorithms in detecting fraudulent financial statements, achieving an F1-score of 0.89.
3.1.2 Unsupervised Learning
Unsupervised learning algorithms are used to identify patterns and anomalies in unlabeled data, making them particularly useful for detecting novel fraud schemes.a) Clustering Algorithms
Clustering techniques such as K-means and DBSCAN group similar data points together, allowing for the identification of outliers that may represent fraudulent activities.
Example application: Bolton and Hand (2001) proposed a peer group analysis method using K-means clustering to detect credit card fraud by identifying accounts that deviate from their peer group's behavior.
b) Anomaly Detection Techniques
Anomaly detection algorithms, such as Isolation Forest and One-Class SVM, are designed to identify data points that deviate significantly from the norm.
Example application: A study by Phua et al. (2010) demonstrated the effectiveness of One-Class SVM in detecting automobile insurance fraud, achieving a true positive rate of 75% with a false positive rate of only 7.5%.
c) Autoencoders for Dimensionality Reduction
Autoencoders are neural networks that learn to compress and reconstruct data. They can be used for dimensionality reduction and anomaly detection in fraud scenarios.
Example application: Paula et al. (2016) used autoencoders to detect credit card fraud, achieving an AUC of 0.95 on a highly imbalanced dataset.
3.1.3 Semi-supervised Learning
Semi-supervised learning techniques leverage both labeled and unlabeled data, which is particularly useful in fraud detection where labeled data may be scarce.a) Label Propagation
Label propagation algorithms spread labels from labeled data points to unlabeled ones based on their proximity in the feature space.
Example application: A study by Lebichot et al. (2019) used a semi-supervised label propagation approach for credit card fraud detection, demonstrating improved performance over supervised methods, especially with limited labeled data.
b) Self-training Algorithms
Self-training involves training a model on labeled data and then using it to predict labels for unlabeled data, iteratively expanding the training set.
Example application: Wang et al. (2018) proposed a self-training approach for online banking fraud detection, showing improved performance over traditional supervised methods, especially in detecting emerging fraud patterns.
3.2 Deep Learning Architectures
Deep learning, a subset of machine learning based on artificial neural networks, has shown remarkable success in fraud detection due to its ability to automatically learn complex patterns from large datasets.3.2.1 Neural Networks
a) Feedforward Neural NetworksFeedforward neural networks, also known as multilayer perceptrons (MLPs), consist of multiple layers of interconnected neurons. They can learn complex non-linear relationships in the data.
Example application: Abroyan and Shumanov (2020) used a deep feedforward neural network for credit card fraud detection, achieving an accuracy of 99.96% on the IEEE-CIS Fraud Detection dataset.
b) Convolutional Neural Networks (CNNs)
While primarily used in image processing, CNNs have found applications in fraud detection, particularly for analyzing spatial and temporal patterns in transaction data.
Example application: Fu et al. (2016) proposed a CNN-based approach for detecting fraudulent financial statements, achieving an accuracy of 86.21%, outperforming traditional machine learning methods.
c) Recurrent Neural Networks (RNNs) and Long Short-Term Memory (LSTM) networks
RNNs and LSTMs are designed to process sequential data, making them particularly useful for analyzing time-series transaction data in fraud detection.
Example application: Jurgovsky et al. (2018) demonstrated that LSTM networks outperformed traditional machine learning methods in credit card fraud detection when considering the sequential nature of transactions, achieving an improvement of up to 19% in AUC.
3.2.2 Graph Neural Networks (GNNs)
GNNs are designed to process data represented as graphs, making them particularly useful for analyzing complex relationships between entities in fraud detection scenarios.a) Graph Convolutional Networks (GCNs)
GCNs apply convolutional operations to graph-structured data, allowing for the analysis of node features and graph topology simultaneously.
Example application: Wang et al. (2019) proposed a GCN-based approach for detecting fraudulent users in online social networks, achieving an F1-score of 0.94, significantly outperforming traditional machine learning methods.
b) GraphSAGE
GraphSAGE is an inductive framework that leverages node feature information to efficiently generate node embeddings for previously unseen data. Example application: Liu et al. (2020) used GraphSAGE for detecting fraudulent accounts in large-scale e-commerce platforms, demonstrating superior performance over traditional graph-based methods.
c) Graph Attention Networks (GATs)
GATs introduce attention mechanisms to graph neural networks, allowing the model to assign different importance to different nodes in a neighborhood.
Example application: Dou et al. (2020) proposed a GAT-based approach for detecting financial fraud in supply chain finance, achieving an F1-score of 0.89 and outperforming other graph-based methods.
3.3 Natural Language Processing (NLP)
NLP techniques are increasingly used in fraud detection to analyze textual data, such as transaction descriptions, customer communications, and social media posts.a) Named Entity Recognition (NER) for document analysis
NER can be used to extract relevant entities (e.g., names, organizations, amounts) from textual data, aiding in the analysis of financial documents and communications.
Example application: Luo et al. (2019) used NER techniques to extract key information from financial reports for fraud detection, improving the accuracy of fraud prediction models by 5%.
b) Sentiment analysis for detecting suspicious communications
Sentiment analysis can be used to identify unusual patterns or emotions in customer communications that may indicate fraudulent activities.
Example application: Goel and Uzuner (2016) demonstrated the effectiveness of sentiment analysis in detecting fraudulent online reviews, achieving an accuracy of 86% in identifying fake reviews.
c) Text classification for categorizing fraudulent patterns
Text classification techniques can be used to categorize transaction descriptions or customer queries into predefined fraud categories.
Example application: Sohony et al. (2018) used text classification techniques to categorize insurance claims descriptions, improving fraud detection accuracy by 12% compared to traditional rule-based systems.
3.4 Computer Vision
Computer vision techniques are increasingly used in fraud detection, particularly for identity verification and document analysis.a) Optical Character Recognition (OCR) for document verification
OCR is used to extract text from images of documents, enabling automated verification of identity documents and financial statements.
Example application: Woodward et al. (2020) demonstrated a 30% reduction in manual document review time by implementing OCR-based automated document verification in a large bank's KYC process.
b) Facial recognition for identity verification
Facial recognition technology is used to verify customer identities during onboarding and high-risk transactions.
Example application: A study by Ratha et al. (2019) showed that implementing facial recognition for identity verification in a major bank reduced identity fraud attempts by 35%.
c) Image anomaly detection for spotting manipulated documents
Advanced computer vision techniques can detect subtle signs of document manipulation, such as altered text or forged signatures.
Example application: Zhang et al. (2021) proposed a deep learning-based approach for detecting manipulated financial documents, achieving a detection accuracy of 98.5% on a dataset of altered bank statements and invoices.
3.5 Ensemble Methods
Ensemble methods combine multiple models to improve overall performance and robustness in fraud detection.a) Bagging
Bagging involves training multiple instances of the same algorithm on different subsets of the data and aggregating their predictions.
Example application: Whitrow et al. (2009) demonstrated that bagged decision trees outperformed individual classifiers in credit card fraud detection, achieving a 28% reduction in financial losses.
b) Boosting
Boosting algorithms, such as AdaBoost and Gradient Boosting, build an ensemble of weak learners sequentially, with each new model focusing on the errors of the previous ones.
Example application: Carmona et al. (2019) showed that a Gradient Boosting ensemble achieved a 15% improvement in AUC compared to individual models in detecting insurance claim fraud.
c) Stacking
Stacking involves training multiple diverse models and then using their outputs as inputs to a meta-model that makes the final prediction.
Example application: Phua et al. (2014) demonstrated that a stacked ensemble of heterogeneous classifiers achieved a 7% improvement in F1-score compared to the best individual model in detecting credit card fraud.
4.1 Real-time Transaction Monitoring
AI models can analyze transactions in real-time, considering multiple factors such as:4.2 Anomaly Detection
Machine learning algorithms can identify unusual patterns that deviate from expected behavior, such as:4.3 Predictive Analytics
AI models can forecast potential fraudulent activities by:4.4 Network Analysis
Graph-based AI techniques can uncover complex fraud rings by:4.5 Behavioral Biometrics
AI can analyze user behavior patterns to create unique profiles, including:Case Studies
5.1 Case Study 1: Large Multinational Bank
A major global bank implemented a deep learning-based fraud detection system, resulting in:5.2 Case Study 2: E-commerce Payment Provider
An online payment processor deployed a graph neural network for transaction analysis, achieving:5.3 Case Study 3: Insurance Company
A large insurer utilized NLP and computer vision for claims fraud detection, leading to:Implementation Framework for Financial Institutions
6.1 Assessment and Planning
6.2 Data Preparation and Integration
6.3 Model Development and Deployment
6.4 Integration with Existing Systems
6.5 Monitoring and Continuous Improvement
Challenges and Ethical Considerations
7.1 Data Privacy and Security
7.2 Bias and Fairness
7.3 Explainability and Interpretability
7.4 Adversarial Attacks
7.5 Regulatory Compliance
Future Trends and Research Directions
8.1 Federated Learning for Privacy-Preserving Fraud Detection
Federated Learning is an emerging technique that allows multiple parties to collaboratively train machine learning models without sharing raw data. In the context of fraud detection, this approach holds significant promise:8.2 Quantum Computing for Enhanced Cryptography
As quantum computers advance, they pose both threats and opportunities for fraud detection and prevention:8.3 Explainable AI (XAI) Techniques
As AI models become more complex, the need for interpretability in fraud detection becomes critical:8.4 AI-Powered Synthetic Data Generation
Synthetic data generation can address data scarcity and privacy concerns in fraud detection:8.5 Integration of Blockchain for Fraud Prevention
Blockchain technology offers unique capabilities that can complement AI in fraud prevention:Conclusion
The integration of AI and ML technologies in fraud detection represents a significant change in perspective in the financial services industry's approach to risk management. This research has demonstrated the significant potential of AI-driven strategies to enhance fraud prevention capabilities, reduce losses, and improve customer experiences. By leveraging advanced techniques such as deep learning, graph neural networks, and behavioral biometrics, financial institutions can stay ahead of evolving fraud threats and maintain trust in the digital financial ecosystem.However, the successful implementation of AI in fraud detection requires careful consideration of technical, ethical, and regulatory challenges. Financial institutions must adopt a holistic approach that combines cutting-edge technology with robust governance frameworks and a commitment to responsible AI practices.
As the field continues to evolve, ongoing research and collaboration between academia, industry, and regulators will be crucial in addressing emerging challenges and unlocking the full potential of AI in fraud detection. By embracing these technologies thoughtfully and responsibly, the financial services sector can create a more secure, efficient, and inclusive digital financial landscape for all stakeholders.
by ML & AI News
4,430 views
Machine Learning Artificial Intelligence News
https://machinelearningartificialintelligence.com
AI & ML
Sign Up for Our Newsletter